TransMon – A large portion of Trust with a side of safety!
Customer experience is the differentiating factor for companies today with each company trying to gain competitive advantage over its contemporaries. Consequently, companies go out of their way to accommodate requests like no-questions-asked return, hassle free membership cancelations, refunds and waivers et al.
While consumers are stoked with convenience with these services, there’s also a small chunk of notorious individuals/entities who exploit it for embezzlement which costs billions (Yes, you read it right) to online retailers. According to a report published by Techradar, losses incurred by online retailers due to fraudulent activities mount up to USD 20 Billion every year. The numbers are equally staggering in India.
Data analytics, Artificial intelligence & machine learning help in reducing the reaction time and take proactive action. While algorithms can proactively take action on accounts, it is important to strike the balance between technology and the human element. Areas of overlap between fraudsters and genuine customers is where the risk is high and the human element is necessary.
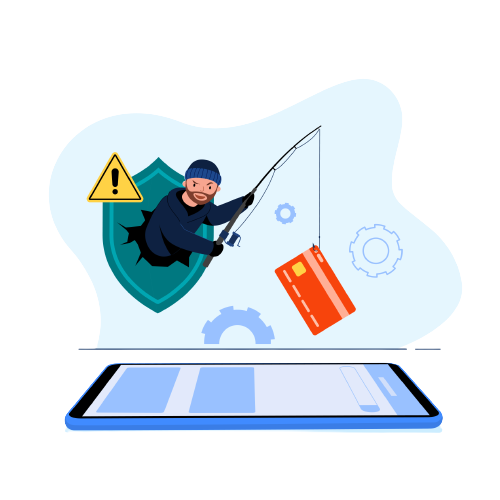
Enter TransMon – India’s leading Quality monitoring tool for all CX touchpoints:
In addition to entire workflow automation and increased productivity resulting in multifold ROI, TransMon also offers detecting and fixing potential fraud triggers. The standard procedure used by TransMon for degerming the fraud landscape on the marketplace is as follows:
Step 1: TransMon through its AI capabilities is able to pre-evaluate 100% population level data of all transactions from all communication channels (Voice & Non-Voice).
Step 2: Identify and (Machine) learn “Risk categories” through Big Data Analytics & Artificial Intelligence algorithms.
Step 3: Trigger ‘investigation’ of possible fraudulent activities to its Quality forensics center of excellence.
Step 4: Quality forensics is a team of trained Quality experts who further scrutinize and filter data to separate genuine cases from fraudulent ones.
Step 5: A comprehensive report is shared on regular basis with the clients with observations and findings.
Step 6: On confirmation from the client, pre-evaluation algorithms are updated for augmenting accuracy and continuously making the system safer.
Cities
Restaurant partners
Delivery partners
orders per day
Use Case
TransMon’s client is an industry leader in Foodtech space operating in over 500+ cities with over 2 Lakh+ delivery partners, 1.5 Lakh+ restaurant partners and clocking in over a million orders per day. Built on the back of robust ML technology and fueled by Terabytes of data every day, the company offers fast, seamless and reliable delivery experience for millions of customers across India.
TransMon is trusted for its CX touchpoint quality monitoring and identifying possible risk categories.
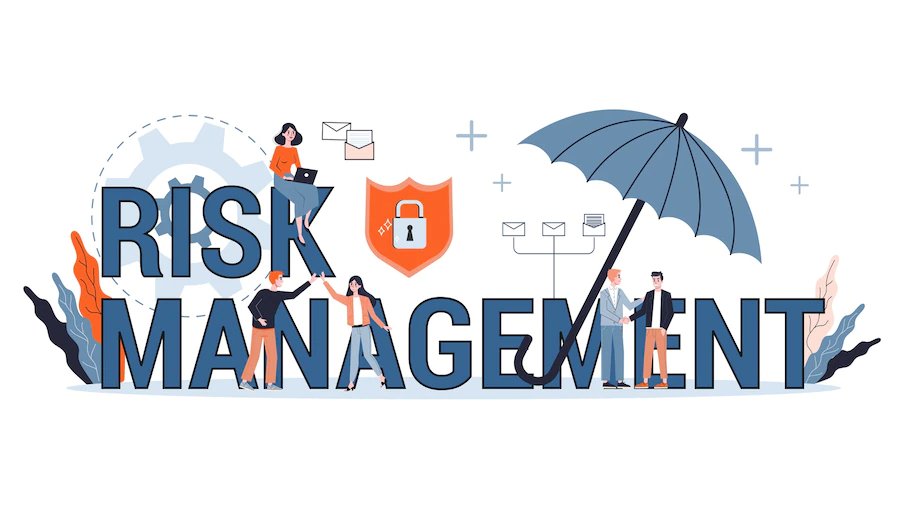
How TransMon realized a loss of over
INR 200,000
within 50 days and potential loss running into millions.
Recent Fraud detection
TransMon’s Text Analytics capabilities pre-evaluated 100% transactions and identified “Multiple D-sat on a single ID” as a risk category.
This was further scrutinized by TransMon Quality Forensic team by mapping Restaurant ID with complaint issue type tagging “Wrong Product Received”.
In a particular case, our Quality Forensic team discovered that there were more than 150 cases reported from 1 restaurant ID in less than 50 days with multiple “Wrong product received” within a single day consistently. On further investigation, it was almost certain that it’s a case of fraudulent activity with realized loss of over INR in 200000 within 50 days and potential loss running into millions.
Observations were shared with the client for the particular case tagged as “Red Alert” category with all data points and evidence. The client investigated it internally and confirmed the fraud.
TransMon contributed in saving millions for its client through its proactive risk assessment. Considering this changing landscape, it is necessary for a business to calculate its profit optimizing point between the cost of anti-fraud review and loss due to fraud.